Are you teaching or joking? Can we ask the robots to help?
Article Date | 29 June, 2022Towards Ai-powered Higher Education
by Shan Wikoon LLB (Hons), LLM, FHEA - Senior Lecturer in Business (LSST Elephant & Castle)
This article explores the need for AI tutor platforms and suggests building and improving such future platforms. The suggestions are based on established learning theories. Therefore, the highlighted points could be very useful for human tutors too.
Come fly with me to the year 2050. My little grandchildren are asking about my job, and I am trying to explain how I lectured to a group of 15 to 30 learners inside a classroom in 2022. They would definitely ask, "Are you joking, grandpa? 'Did you actually try to teach that many students in one go?' I do not doubt that they will laugh at my teaching method.
Many Higher Education (HE) institutions in the UK are now committed to widening participation initiatives (DBIS, 2014). Under the widening participation, thousands of mature students who had no access to the UK education system enter higher education now. Most of them lack study skills and IT skills to pursue degree-level courses. They have limited time to improve those skills at a fast pace as they juggle work, family, and study life. Meanwhile, some students who have already acquired knowledge and skills through work experiences demand fast-paced delivery of lessons comprising full of rich learning activities. We struggle to address their needs while supporting students with special needs.
Among full-time undergraduates enrolled in 2020-21, 14.8% reported having a disability (OfS, 2022). This could be a physical impairment or mobility issues, dyslexia, dyspraxia or AD(H)D, Asperger's syndrome/other autistic spectrum disorder, depression, schizophrenia or anxiety disorder, deaf or serious hearing impairment, blind or serious visual impairment, etc.
Furthermore, international students in HE create another challenge due to their lack of English language skills and cultural differences. The UK government planned to increase education exports to £35 billion per year and increase the number of international higher education (HE) students studying in the UK to 600,000 annually by 2030 (DITDE, 2021). 'High participation in higher education is becoming near-universal across the world' (Marginson, 2016). They all are in great need of flexibility in the pace, place and mode of learning. Presently, we try to fit all those students in one classroom and teach them all together.
Now, can you understand why my grandkids would laugh at me?
'if each child is unique, and each requires a specific pedagogical approach appropriate to him or her and no other, the construction of an all-embracing pedagogy or general principles of teaching become an impossibility' (Simon, 1999, p42)
How can we meet the needs of such a diverse learner population and create a truly inclusive and high-quality learning environment at a low cost?
Currently, we are teaching groups of students in big classrooms just because we do not have any other reasonable alternative. In ideal circumstances, one student should be taught by one instructor at a time that is convenient for the student.
Need for one-to-one learning:
In 1984, Benjamin Bloom identified that the ideal learning model is one-to-one tutoring with a success rate of two sigma. Because one tutor facilitates one student by providing tailored, adaptive contents until the student achieve the learning outcomes through frequent corrective feedback and motivation for progression (Bloom, 1984). The student had an opportunity to review and relearn the contents as many times as required. When one teacher teaches 30 students, such effects cannot be achieved. Bloom's study proved that if a class of 30 students underperformed, that is mainly due to the wrong teaching method and not due to the students' weaknesses. However, as we can not provide one tutor for one student, the success rate of two sigma can not achieve. Bloom labelled this challenge as the "Two sigma problem".
No institution or country can provide such individual tutoring to every student with human tutors at the moment. We know we cannot be perfect teachers, but we try our best to reach perfection. How? We try to iron out the imperfections/ineffectiveness of our current group teaching methods by deploying various tools, models and concepts such as
- Student-centered teaching
- Differentiated teaching
- Flipped learning
- Active learning methods
- Using virtual learning environments and other academic and pastoral support services
- Andragogical practices for adult learners
In other words, with a bucket with many holes, I try to carry water. The above remedies are a few patches applied to my bucket. But water leaks further through other holes.

So what is the solution? Google driverless cars are now driving themselves in the USA. Google, as well as many other car companies, have created AI drivers.
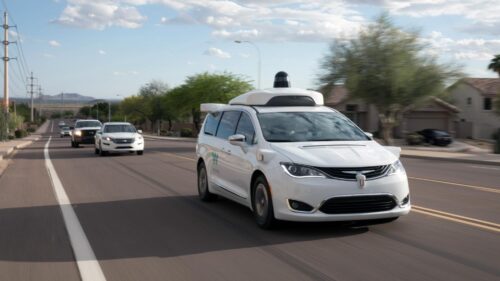
Can we also create an AI teacher for our students?
AI: "An agent is anything that can be viewed as perceiving its environment through sensors and acting upon that environment through actuators" (Russel & Norvig, 2010, p. 34). (This agent nearly replicates human intelligence but acts independently from the human commands.)
Towards a personalised learning experience using AI
Maguire, Dale and Pauli (2020) promoted the vision for 2030 by stating, 'Students benefit from a personalised learning experience. The widespread adoption of artificial intelligence (AI) provides a learning experience that effortlessly melds the preferences and needs of the individual learner.’
Dedicated online courses with rich content have been used for decades (Example: Open University UK). Many companies are now using the power of artificial intelligence to fine-tune the right content for individual learners' needs and learning styles. (Zawacki-Richter et al., 2019). For example, Squirrel AI (2021) claims that their AI tutor can identify and improve around 3000 weaknesses of a student in a single subject, which is an impossible task for a human teacher. It is encouraging that Google expands Google Cloud AI to power an interactive tutor platform for individualised learning (Butschi, 2021).
In other words, they are creating an AI teacher for every student who customises content for the individual learner according to the learners’ specific learning style, abilities, needs and pace. “Adaptive learning…has the potential to transform higher education by improving the quality of the educational experience through personalisation” (Liu et al., 2017).
Adaptive Learning Technologies: “Dynamically adjust to the level or type of course content based on an individual’s abilities or skill attainment, in ways that accelerate a learner’s performance with both automated and instructor interventions.” - (Pugliese, 2016)
The effectiveness of such Intelligent Tutoring Systems (ITS) is proven by a few studies but with a caveat. Such ITS should apply appropriate pedagogical strategies to get significant results (Steenbergen-Hu and Saiying, 2014). Poorly designed virtual learning environments could drive the learner to a state of confusion (Hara & Kling, 2000).
What should essential pedagogical strategies be applied to create an effective AI Tutor?
The AI Tutor platform should:
Create a one-to-one learning platform for every student
Deliver the content around the clock on-demand and online
Conduct diagnostic tests on learner's prior knowledge and abilities
Receive and provide comprehensive feedback
Promote active learning
Deliver the content and learning activities customised to the learner's learning style, abilities, needs, and pace
Motivate learning and completion of the assessment tasks
Facilitates collaborative learning as much as possible
Constantly assess the learners' progress and remedy the weaknesses until the learner achieves mastery and the learning outcomes
Only direct the learner to master the knowledge contents or set of skills that the learner indeed lacks
Signpost the student to a human tutor if additional support is needed
Show empathy, tenderness and enthusiasm
Provide a friendly user interface and experience to both student and the content creators
Let’s deep dive into the pedagogical and theoretical aspects of the above factors.
The student-centred learning
The student-centred learning model is widely accepted within the higher education sector. Edwards supported the model by stating, 'Waste in human and educational resources is reduced as it suggested learners no longer have to learn what they already know or can do, nor what they are uninterested in’ (2001, p37). The AI Tutor platform will be designed in a manner that AI will only direct the learner to master the knowledge or skills that the learner truly lacks. Lea et al. clarified seven attributes of student-centred learning (2003, p.322). The AI Tutor should build around those attributes, especially the reliance on active rather than passive learning, emphasising deep understanding and increased learner accountability.
A truly personalised learning platform should satisfy the learner's psychological need for competence, autonomy, and social relatedness. Self-determination theory underlines the importance of those psychological needs (Ryan and Deci 2002). Because AI Tutor facilitates choices, volitional engagement, and a sense of relevance, it increases the learner's intrinsic motivation and, subsequently, high-quality learning.
Effective assessment and adaptive teaching
Any AI Tutor platform should perfect four aspects to assure effectiveness (Ohlsson, 1986). Firstly, it should correctly diagnose the learner's cognitive status, learning styles and capabilities. Then, subject matter analysis and appropriate teaching tactics and strategies should be applied. In this sense, conducting diagnostic tests is very important before the beginning of the teaching. When the teaching begins, any AI Tutor should be able to read every second of the learner's mind. Ohlsson argued that if ITS solely relies on the errors made by the learners in the past and cannot sense their current cognitive status of them, then such ITS becomes a limited remedial tutoring system (1986, p. 318). The human tutor may not remember every mistake made by the learner, but humans are more capable of understanding the learners' current mindset and motivation level through body language. Don't they? Building an adaptive AI Tutor that can capture the current cognitive status is challenging; it is harder to train such AI for applying the right teaching tactics to each scenario. E.g. explaining the same topic in a different style when the student is confused, cracking a joke when the student is bored, delivering motivational talks when the student is procrastinating. Effective technological solutions should be built for the above matters, but those solutions should not be expensive or too complex such as a wearing brain-scanning headset. Hughes (2011) stressed that current criteria-referenced and standards-based assessment is no longer fit for purpose. She argued that combining the current assessment regime with ipsative assessment can increase the learner's intrinsic motivation, self-esteem, and performance. The most significant positive factor of the AI Tutor platform would be its ability to facilitate ipsative assessment and feedback. Hughes and Harris (n.d.) wrote, "Assessment is key to retention". Ipsative feedback motivates the learner by informing them of their progress and supporting them in remedying their weakness at the right time (Hughes, 2017). The AI Tutor will continuously receive and record feedback on the learner's progress which will never be forgotten but applied to suggest the learner's next move within the curriculum.
Effective Feedback
The learners cannot understand whether and how to achieve the learning outcomes unless they receive frequent, timely, developmental feedback and feedforward. (Nicol & Macfarlane‐Dick's, 2007). However, most HE educators currently fail to provide such effective feedback due to time constraints (Henderson, Ryan and Phillips, 2019). The AI Tutor would provide adequate, timely feedback for a large number of students at a relatively low cost (Kulik and Fletcher, 2015). The AI Tutor can receive data on the student's progress, memorise them, and act timely. The robots will not feel tired and take breaks.
Active learning needs
Baume and Scanlon (2018) identified seven principles of effective learning. Principles 4, 5 and 6 emphasise that learning should be an active process; learners should spend lots of time on the tasks such as collaborative activities, doing relevant things and practising because humans learn through doing things and then reflecting on what they have done and achieved. Schindlholzer (2016) proposed a three-part model of problem-based learning (PBL), simulation and immersion where the learner can apply their knowledge in a safe environment and learn through repeated failures and successes. Gamification in higher education is also becoming popular now (Sailer and Homner, 2020). Gee (2003) highlighted that the interactions in a game adapt to the skills and actions of the individual, effectively creating one of the primary motivational factors to play. Any education game should be equipped with the same adaptation ability (Conati, 2002). The AI Tutor can easily implement all the above with adequate student support.
Motivational AI tutor
High drop-out rates are common in distance learning courses (Park & Choi, 2009), such as the courses of Open University in the UK, where 38% of e-learners leave before submitting their first assignment (Simpson, 2004). Therefore, the strategies for engaging and motivating learners should be implemented. More humanoid interfaces with human faces and conversations are AI tutors' essential parts.
The studies proved that the human tutor is still more effective than many ITS, mainly because it could motivate the learner better (Vanlehn, 2011). Most of the previous ITSs were designed not to replace the human tutor but to provide more proactive personal guidance to the students (Rovira, Puertas, & Igual, 2017). Therefore, qualified lecturers will regularly oversee the data generated by the AI Tutor and intervene in the process when underperforming or non-engaging students are detected. The learners should be able to signpost themselves to human tutors without obstacles via the AI Tutor.
Collaborative Learning
Even though personalised learning platforms are essential, the value of collaborative learning can not be ignored. It is one of the core teaching practices for UK HE according to the UK Quality Code (QAA, 2018). Constructivism emphasises interactive communication and collaborative participation to ignite effective learning (Wallace, 2003). Many social constructivists highlighted the value of collaborative learning, especially among adult learners who can share rich life experiences (Vygotsky,1978), (Rogers, 1994). According to the social presence theory, learners do need psychological connections with others (tutors and peers). Kim (2011) identified five social presence theory components: affective connectedness, open communication, collectiveness, mutual attention, empathy, and interdependent support. All those components should be able to be incorporated into AI Tutor creatively. E.g. to show empathy, motivational messages can be displayed when the student could not score good points on a quiz. Then, the adaptive technology will present more manageable tasks until the student rebuilds the confidence. The learning system can be constructed to mimic the interaction of a caring tutor. Synchronised watching (watch party) or an option to add comments can be facilitated. Communication with the peers who are using the AI Tutor can be improved by using a display board showing the live participants and their progress status (with their approval). Asynchronous collaborative activities can be added to the AI Tutor, enabling the participants to engage whenever possible.
User interface (UI) and user experience (UX)
Building an AI-powered tutor involves considerable cost and time unless an established tech company provides a plug-and-play platform (Welham, 2008). The best practice would be to build the base of AI tutors by the tech companies in a way that the educators can enrich and adapt the platform according to their needs without facing many technical hurdles. There should be two user interfaces, one for the human teachers who create contents and one for the students. While supporting the teachers in finding appropriate content, AI should be able to serve diverse student needs, including special learning needs.
How soon can we interact with a truly effective AI tutor? Keep your fingers crossed.
.
References (In Harvard style):
Baume, D. and Scanlon, E. (2018) ‘Learning: what research can tell us about how and why learning happens’ in Luckin, R. (ed.) Enhancing learning and teaching with technology: what the research says. London: UCL IOE Press, pp. 2–13.
Bloom, B. (1984) ‘The 2 Sigma Problem: The Search for Methods of Group Instruction as Effective as One-to-One Tutoring’, Educational Researcher, 13(6), pp. 4–16. https://doi.org/10.3102%2F0013189X013006004
Butschi, S. (2021) New Google Cloud Student Success Services help educators scale individualised learning. Available at: https://cloud.google.com/blog/topics/public-sector/new-google-cloud-student-success-services-help-educators-scale-individualized-learning [Accessed 20 December 2021].
Conati, C. (2002) ‘Probabilistic assessment of user’s emotions in educational games’. Applied Artificial Intelligence, 16, pp. 555–575. https://doi.org/10.1080/08839510290030390.
DBIS (2014) National Strategy for Access and Student Success in Higher Education. Available: https://www.gov.uk/government/publications/national-strategy-for-access-and-student-success (Accessed: 16 January 2022).
DITDE -Department for International Trade and Department for Education (2021) Policy paper: International Education Strategy: 2021 update. Available at: https://www.gov.uk/government/publications/international-education-strategy-2021-update (Accessed: 14 November 2021).
Edwards, R. (2001) ‘Meeting individual learner needs: power, subject, subjection’ in C. Paechter, et al. (Eds.), Knowledge, Power and Learning. London: SAGE. pp. 37-46.
Gee, J. P. (2003) What video games have to teach us about learning and literacy. New York: Palgrave Macmillan. https://doi.org/10.1145/950566.950595.
Hara, N., & Kling, R. (2003). ‘Students’ Distress with a Web-based Distance Education Course: An Ethnographic Study of Participants' Experiences’. The Turkish Online Journal of Distance Education, 4.
Henderson, M., Ryan, T. & Phillips, M. (2019) ‘The challenges of feedback in higher education, Assessment & Evaluation’, Higher Education, 44 (8), pp. 1237-1252, https://doi.org/10.1080/02602938.2019.1599815.
Hughes, G. (2011) ‘Towards a personal best: a case for introducing ipsative assessment in higher education’, Studies in Higher Education, 36(3), pp. 353–367. https://doi.org/10.1080/03075079.2010.486859.
Hughes, G. and J. Harris (forthcoming) ‘Retention and Success: Approaches and Tools for Making a Difference’. (London: University of London).
Hughes. G. (2017) Ipsative Assessment and Personal Learning Gain: Exploring international case studies, Basingstoke: Palgrave Macmillan.
Kim, J. (2011) ‘Developing an instrument to measure social presence in distance higher education’, British Journal of Educational Technology, 42(5), pp. 763–777. https://doi.org/10.1111/j.1467-8535.2010.01107.x.
Kulik, J. A. and Fletcher, J. D. (2016) ‘Effectiveness of Intelligent Tutoring Systems: A Meta-Analytic Review’, Review of Educational Research, 86(1), pp. 42–78. https://doi.org/10.3102%2F0034654315581420.
Lea et al. (2003). Higher Education Students' Attitudes to Student-Centred Learning: Beyond 'educational bulimia'. Studies in Higher Education. 28(3), pp. 321–334
Liu, Min & Kang, Jina & Zou, Wenting & Lee, Hye Yeon & Pan, Zilong & Corliss, Stephanie (2017) Using Data to Understand How to Better Design Adaptive Learning. Technology, Knowledge and Learning. Available at: https://link.springer.com/article/10.1007/s10758-017-9326-z (Accessed 27 February 2022)
Maguire, D., Dale, L. and Pauli, M. (2020) Learning and teaching reimagined A new dawn for higher education? Available at: https://repository.jisc.ac.uk/8150/1/learning-and-teaching-reimagined-a-new-dawn-for-higher-education.pdf [Accessed 20 December 2021].
Marginson, S. (2016) 'The worldwide trend to high participation higher education: dynamics of social stratification in inclusive systems', Higher Education, 72, pp.413–434.
Nicol, D. & Macfarlane‐Dick, D. (2007) ‘Formative assessment and self‐regulated learning: a model and seven principles of good feedback practise’, Studies in Higher Education, 31(2). pp. 199-218, https://doi.org/10.1080/03075070600572090
OfS (2022) Equality, diversity and student characteristics data. Available at: https://www.officeforstudents.org.uk/data-and-analysis/equality-diversity-and-student-characteristics-data (Accessed 20 June 2022)
Ohlsson, S. (1986) ‘Some principles of intelligent tutoring’. Instructional Science, 14, pp. 293-326.
Park, J., & Choi, H. (2009) ‘Factors Influencing Adult Learners' Decision to Drop Out or Persist in Online Learning’. J. Educ. Technol. Soc., 12, pp. 207-217.
Pugliese L (2016) Adaptive learning systems: surviving the storm. EDUCAUSE Review. Available at: https://er.educause.edu/articles/2016/10/adaptive-learning-systems-surviving-the-storm (Accessed 27 February 2022)
QAA (2018) UK QUALITY CODE FOR HIGHER EDUCATION. Available at: https://www.qaa.ac.uk/quality-code (Accessed 27 February 2022).
Rogers, C. (1994) Freedom to learn for the 80's. 3rd edition. New York: Merrill.
Rovira, S., Puertas, E., & Igual, L. (2017) ‘Data-driven system to predict academic grades and dropout.’ PLoS One, 12(2), pp. 1–21. https://doi.org/10.1371/journal.pone.0171207
Russel, S., and Norvig, P. (2010) Artificial intelligence - a modern approach. New Jersey: Pearson Education.
Pugliese, L., (2016) Adaptive Learning Systems: Surviving the Storm. Available at: https://er.educause.edu/articles/2016/10/adaptive-learning-systems-surviving-the-storm (Accessed 16 January 2022).
Ryan, R. M., & Deci, E. L. (2002). ‘Overview of self-determination theory: An organismic-dialectical perspective’. In E. L. Deci & R. M. Ryan (Eds.), Handbook of Self-determination Research, Rochester, NY: University of Rochester Press, pp. 3–33.
Sailer, M. and Homner, L. (2020) ‘The Gamification of Learning: a Meta-analysis’, Educational Psychology Review, 32(1), pp. 77–112. https://doi.org/10.1007/s10648-019-09498-w
Schindlholzer, B. (2016) Artificial intelligence & the future of education systems | Bernhard Schindlholzer | TEDxFHKufstein. 4 Aug. Available at: https://youtu.be/ZdHhs-I9FVo (Accessed 27 February 2022).
Simon, B. (1999) ‘Why no pedagogy in England?’ In J. Leach and B. Moon (Eds.), Learners and Pedagogy. London: Sage Publications.
Simpson, O. (2004) ‘Student Retention and the course choice process: the UK Open University experience.’ Journal of Access Policy and Practice, 2, pp. 44-58
Squirrel AI (2019) How AI Make Personalised Education Affordable to Every Family in China. Available: https://www.ai-expo.net/global/wp-content/uploads/2019/04/1430_Wei-Cui_Squirrel-AI-Learning_AI-Technology-Solutions.pdf (Accessed: 14 November 2021).
Squirrel AI (2021) Intelligent Adaptive Learning System (IALS) Available: http://squirrelai.com/product/ials (Accessed: 14 November 2021).
Steenbergen-Hu, S. & COOPER, H. (2014) ‘A meta-analysis of the effectiveness of intelligent tutoring systems on college students’ academic learning’. Journal of Educational Psychology, 106 (2), pp. 331-347.
Vanlehn, K. (2011) ‘The Relative Effectiveness of Human Tutoring, Intelligent Tutoring Systems, and Other Tutoring Systems". Educational psychologist (0046-1520), 46 (4), p. 197.
Vygotsky, L. (1978) Mind in Society. London: Harvard University Press.
Wallace, R. (2003) ‘Online learning in higher education: a review of research on interactions among teachers and students’. Education, Communication & Information, 3 (2), pp. 241-280.
Welham, D. (2008) ‘AI in training (1980–2000): Foundation for the future or misplaced optimism?’ British Journal of Educational Technology, 39(2), pp. 287–303. https://doi.org/10.1111/j.1467-8535.2008.00818.x
Zawacki-Richter, O., et al. (2019) ‘Systematic review of research on artificial intelligence applications in higher education – where are the educators?’. International Journal of Educational Technology in Higher Education, 16 (39) https://doi.org/10.1186/s41239-019-0171-0
Sharing is caring!